Optimizing all the variables related to comminution and separation requires sophisticated control strategies.
By Devin Marshman, Spartan Controls and David Harrach, Emerson
Ore processing operations connected with mining sites are a critical but costly step in the larger effort to produce refined metals. Regardless of the final purification process, ore must be prepared to meet refiner composition specifications, create optimal particle size, support preliminary separation, and impart other characteristics necessary to ensure efficient final separation, whether by chemical means or traditional smelting. Steps can involve grinding, screening, thickening, flotation, and others to achieve the desired intermediate product.
These steps depend on automated process control to maximize recovery of on-spec product in a cost-effective manner. Grinding operations are generally the most energy intensive steps of a processing plant, often consuming up to 10 kilowatt hours per ton of ore processed. Selecting the most effective control strategies for this and other processing equipment is the subject of much discussion at many mining companies given the enormous stakes involved.
The ability to shave even a few percentage points off total energy consumption per ton can result in huge increases to a facility’s bottom line, along with substantial improvements to efficiency and sustainability.
Advanced process control (APC) strategies are becoming increasingly critical to ore processors, but there are different approaches under this umbrella. Process designers must consider carefully which to adopt, whether from the most current APC options, or more traditional approaches.
Understanding process control
To put this discussion into context, it helps to think about some basic concepts of process control automation, and how it can be applied in ore processing applications.
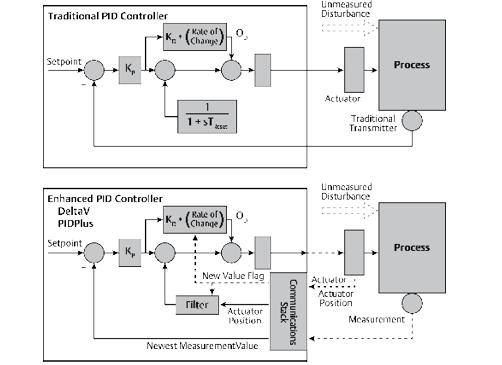
can’t handle all the complex operations of ore processing.
Regulatory Control – This traditional method, which relies primarily on PID-based loop control, is the most basic building block of automation (Figure 1), and there will undoubtedly be many small controllers throughout a facility using this approach. A sensor measures a process variable and compares it to a setpoint. If the variable deviates from the setpoint, it adjusts an actuator to bring it into line. For example, to control liquid flow, a flow meter provides the process variable, and a control valve is the actuator, changing its position as needed to bring the actual flow into line with the setpoint. The system response can be tailored by adjusting the proportional, integral and derivative parameters – but this method of control is often not sufficient.
This approach can be very effective for appropriate applications, but it has serious limitations. First, it can only handle one thing at a time because each process variable must be paired with a single and distinct point of actuation. Where there are multiple variables related to the same application, designers may try multiple loops in sequence, in parallel, or loops within loops, but these can get complex and difficult to control.
Second, regulatory control is reactive in nature, making an adjustment only when the variable moves out of range. It can’t anticipate a change, so if the process is slow moving, it may not be able to respond quickly enough to avoid an excursion into an undesirable range.
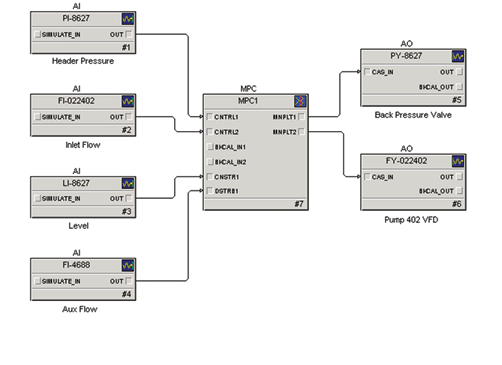
regulatory control, but it still has critical limitations.
Model Predictive Control – Model predictive control (MPC) takes a different approach, and it can be applied to simple and complex multi-variable challenges. It creates a mathematical representation of the process (Figure 2), quantifying the various inputs necessary to achieve one or more desired outputs. For example, reaching a given ball mill load might require specific inputs of feedstock flow, water flow, mill speed and other variables. The model will determine what all those inputs must be to achieve the specified output, and then adjust those inputs accordingly.
This approach has gained popularity for ore processing because it can handle complex operations where there are multiple potential variables. Under operating conditions where the process is generally stable and changes are gradual, MPC can be effective, so it should be in a designer’s toolbox. However, it also has potential pitfalls.
First, it is only as good as its model. If stoichiometric or physical relationships are not characterized correctly, the controller will not respond correctly and may drive the process out of the desirable range. Once this is recognized, the model can be adjusted, but it is not always easy to recognize where the problem lies. Any time there is a change in the equipment or process, including a critical feedstock characteristic, the model must also be adjusted, complicating what would normally be considered a simple change. Some of the most frequent adjustments, such as feedstock characteristics, could be built into the operator’s human machine interface, but adjusting for equipment changes might be more difficult. Non-linear aspects of the process make this particularly challenging toward the high or low extremes of any variable.
Second, MPC is a finite-horizon controller, which means that its goal is smoothly and gradually bringing the process to its optimal setpoints at some given point in the future. In doing so, MPC avoids large or sudden changes to its points of actuation, preferring to gradually ramp them instead. Without extremely specialized tuning, MPC does not have the same “proportional kick” in response to upsets or setpoint changes that you can achieve with PID.
In practical operation, MPC is best where the controller can move the process to its comfortable operating level and keep it there. But where there are frequent and potentially drastic changes caused by an upset, such as in a SAG mill, MPC may not be able to respond quickly enough. Unstable situations call for a strong proportional action, where the controller responds to a rapid change with a rapid and strong correction. MPC can struggle in such situations.
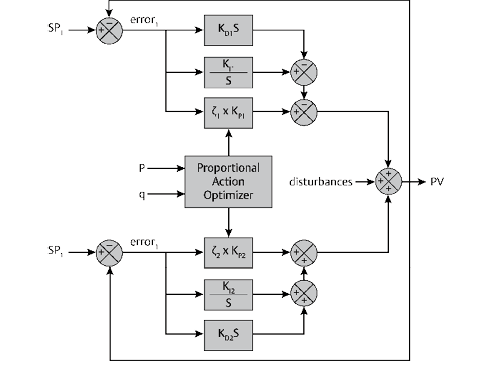
benefits of traditional regulatory control.
Integrated Quadratic Control – Integrated quadratic control (IQC) is a newer method of closed-loop control, and its use is growing rapidly for ore processing applications. It is a single-input, multi-output controller (Figure 3), that uses velocity-form quadratic optimization to split the output signal. This allows it to control multiple functions simultaneously, even to the point of adjusting and optimizing its strategy where it has multiple options to achieve the desired setpoint.
For each control output, IQC defines two sets of operating limits: one normal high and low limit, and one extreme high and low limit. It then characterizes the process by determining how far it can push the controller outputs within the normal operating range to meet the controller setpoint, up until the controllable variables are saturated.
However, if all control outputs are saturated and IQC cannot reach setpoint within the normal operating range, it will expand the limits to the extreme values. The extreme values are defined as hard limits, and they are typically only used during upset recovery or abnormal operation. Controller gains are automatically modified in this extreme region to address the nonlinear behavior of abnormal operation. IQC can also automatically adjust the proportionality of its control efforts to chase major setpoint adjustments or process upsets quickly, allowing it to reoptimize outputs with minimal lag time.
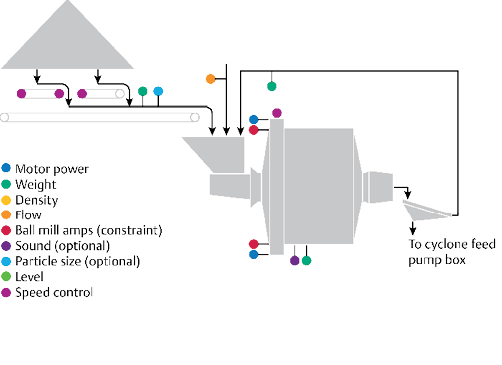
Applying IQC
IQC can be applied in many processes, but there are two areas of ore processing where it has been particularly beneficial: SAG mills and flotation separators.
SAG mills (Figure 4) are a critical step in the process, but for many ore processors, they are also major headaches:
- A frequent bottleneck, responsible for constraining overall throughput.
- A facility’s biggest energy consumer.
- Difficult to control due to feed variability.
- A frequent source of trips, originating from multiple causes, which can interrupt production.
- Costly to maintain with expensive liners that wear rapidly when misused.
Operators must monitor, and sometimes adjust, a long list of variables, including:
- Motor power, which might be constrained by available amperage limits.
- Mill speed.
- Input flow rate and feedstock characteristics.
- Recycled product weight and characteristics.
- Total weight and density of product in the mill at any given time.
- Water input.
- Downstream inventory and processing capacity.
These must all be balanced carefully to ensure maximum throughput with optimized particle size, while avoiding, or at least accommodating, sudden feed-rate changes. Operators must also monitor power consumption and operate in a way that maximizes liner life. Clearly, balancing all those variables calls for very responsive and sophisticated process control, which is possible using IQC.
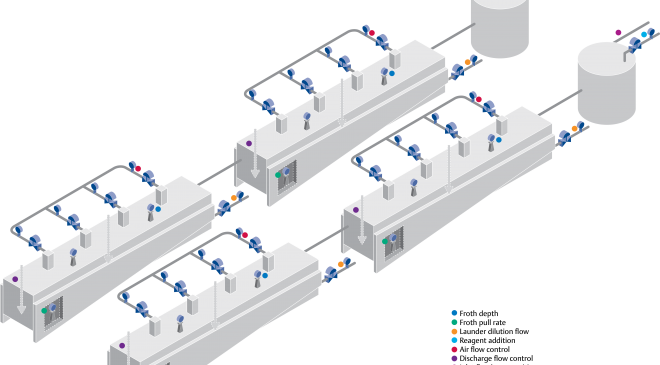
Flotation separators (Figure 5) present challenges related to their multiple-input and multiple-output variables, which must be controlled simultaneously. This is especially difficult because the pull rate from each cell along the flotation bank may not have its own flow rate measurement. IQC’s multi-output optimization within a defined normal operating region addresses this problem by ensuring that all cells move together, rather than compensating for flow rate changes by throttling a single cell.
Immediate response to feed changes is another critical aspect of effective flotation operation. Product recovery rates can change significantly during upsets if cell air, level, and reagent addition are not also adjusted to match. Even temporary upsets to the flotation cell can quickly lead to off-grade product, contaminated tailings, or sanded-out cells.
The IQC controller must automatically coordinate multiple variables across each cell within a bank to maintain safe and stable operating conditions, including cell level and pull rate, reagent (e.g., flocculant) addition rates, and air injection rates.
By coordinating the above variables to maintain ideal concentrate pull rates, IQC can effectively control flotation under a wide range of operating conditions. Once this has been achieved, supervisory optimization can be added to coordinate target pull rates on various flotation banks at the site to maximize net product recovery based on final product grade and available processing capacity.
Approaching APC upgrades
The two applications just discussed are not the only areas that can benefit from IQC. Others may not be as complex, but they can also be critical to improving overall facility performance. The list can include thickeners, clarifiers, pH control, pump-box level control, grade control, and multi-pump applications. When making evaluations, a company must consider the criticality and complexity of each, and then apply the correct control strategy.
Many facilities look at the range of applications individually and select separate controllers for each, trying to tailor the solutions, one-by-one. This can work, but it creates a fragmented environment requiring extensive manual coordination. A more practical alternative is to choose one large-scale automation host system that can support multiple control strategies and coordinating the entire operation.
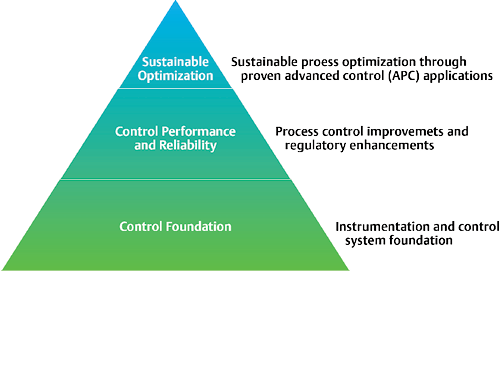
manufacturing facility to increase efficiency.
APC and sustainability
This approach is especially important as mining companies, along with virtually every other industry, are coming under increasing pressure to meet sustainability goals. How these are defined and realized vary from company to company, but generally involve reductions of carbon footprint, manifested as lower energy consumption overall and reduced fossil fuel use. As a practical matter, companies can make significant advances via better internal energy management and making processes more energy efficient. Effective control helps accomplish both.
Successful sustainability improvements over time generally come as the result of tightening up the entire operation, often starting with improved process control strategy (Figure 6). Effective control, especially when APC is in use, depends on top-notch, well-maintained instrumentation. Without reliable process measurements and effective actuators, APC can’t tame complex operations.
Comminution processes are especially important in this effort since they represent the largest single energy use in the entire mining process. It’s common to find comminution accounting for more than 50% of overall energy consumption and 10% of total production cost, so the ability to move the cost needle in this area has enormous impact.
Mining operations can support sustainability efforts by working with a qualified partner organization capable of understanding the full scope of such a program, with the experience needed to guide planning and implementation. With effective strategy is combined with well-chosen automation technologies, improved sustainability will result, along with increased profitability.
Editor’s note: All figures courtesy of Emerson.