Maintenance Represents A Significant Cost To Mining Companies. It’s Time To Do It Smarter.
By Jonathan Rowland
In December 2017, global accountancy and business advisory company BDO released a series of near-term predictions for the mining industry. One of their expectations for the U.S. mining industry foresaw spending on information technology (IT) growing to 10% of revenue by 2020 from a mere 1% in 2015.
In a follow-up assessment of its predictions released two years later,1 BDO noted the industry was on track to fulfill that prediction with “many mining companies […] adopting predictive maintenance and mine management software that allows for automation.” It is the first of these that is the focus of this article.
It’s Not All About Driverless Trucks!
To effectively compete in today’s international marketplace, mining companies must wring every dollar from their operating budget. Maintenance may not be the flashiest of subjects, but it is an area in which the adoption of digital and IIoT technologies can result in major savings. After all, maintenance costs in the mining industry account for 30%-50% of operating expenditures.2 Despite this, many mining companies are yet to catch up with best practices in maintenance strategy.
“Most mining companies still operate on a preventative or planned maintenance strategy, resulting in unplanned downtime and preventable, costly repairs,” said Martin Provencher, industry principal for Mining, Metals and Materials at AVEVA (formerly OSIsoft).3 Moreover, according to a 2021 paper from Bently Nevada,4 a company that specializes in condition monitoring and machine asset management, 60%-80% of equipment problems in mining operations stem from “poor, untimely or incorrect maintenance”.
According to Bently Nevada, “a majority of the mining industry uses rule-based SCADA monitoring systems that cannot identify potential failures when manually set thresholds are not crossed. As a result, more than 80% of mines are unable to quantify the complete cost of downtime due to the various complexities involved. While putting a precise figure to this cost is difficult, unplanned downtime can easily run into hundreds of thousands of dollars per year [… and] companies not using a proactive maintenance approach face a significant amount of unplanned downtime that costs two to five times more than planned maintenance expenditure.”
The opportunity for improvement is therefore significant. As Provencher noted, “moving from preventive to predictive maintenance can enable mining companies to improve their overall equipment effectiveness (OEE) from 50%-75% to 90% or more.”
Five Approaches to Maintenance
When it comes to maintenance there are several strategies available: reactive, preventative, condition-based, predictive and prescriptive (Figure 1).5 Reactive maintenance runs equipment to breaking before fixing or replacing it. Although this approach ensures you get the longest possible life out of your equipment, downtime is unpredictable and can have larger impacts on productivity.
Preventative (or planned) maintenance improves reliability by replacing parts on a regular cycle, before they break. However, as Deloitte explained in a 2017 paper,6 “it can be difficult to determine how often a machine should be taken offline to be serviced, as well as weigh the risks of lost production time against those of potential breakdown. Traditionally, this dilemma forced most maintenance organizations into a trade-off situation where they had to choose between maximizing the useful life of a part at the risk of machine downtime, attempting to maximize uptime through early replacement of potentially good parts, or, in some cases, using past experience to try to anticipate when breakdowns might occur and addressing them proactively.”
Condition-based maintenance tries to take the guesswork out of that dilemma and is the “first step towards adopting a future-facing maintenance strategy,” according to OSIsoft.7 It uses real-time data, collected from sensors, operator observations etc., to identify when as asset’s performance falls to an unacceptable level.
“By observing the state of an asset […] maintenance professionals can identify when an asset is about to fail or has failed,” explained maintenance management software provider, added FasTrak in a 2020 blog.8 “With condition-based maintenance, work is performed only when needed in response to the asset’s real condition, preventing unnecessary maintenance tasks.”
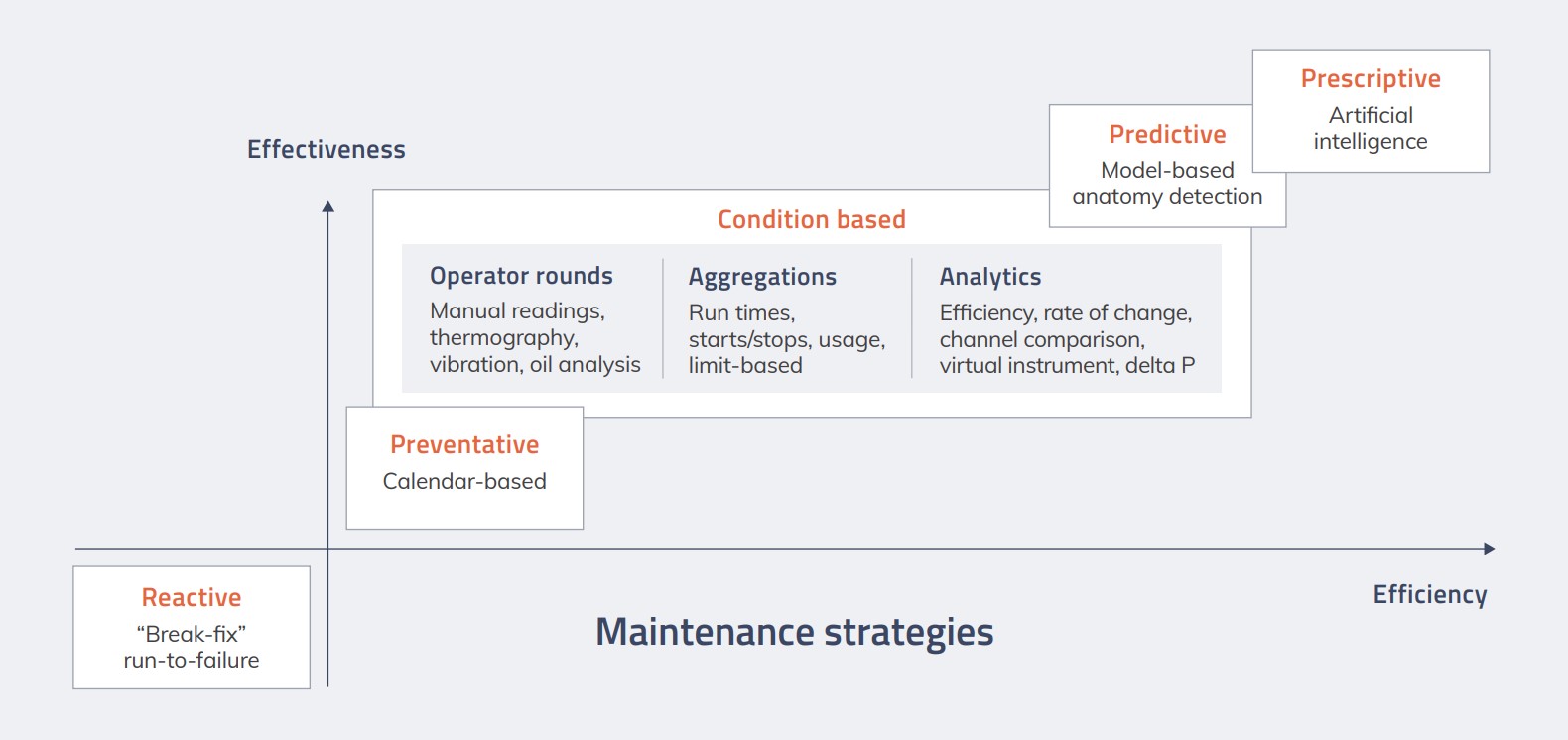
Which brings us to predictive maintenance. This takes the real-time nature of condition-based maintenance and moves it into the future, using model-based anomaly detection to predict when a piece of equipment is going to fail. As FasTrak continued, “the key difference is timing – what is the condition of an asset right not (condition-based maintenance) versus what might the condition of an asset be in the future (predictive maintenance).”
The benefit of predictive maintenance is that it allows maintenance teams to create an optimized maintenance schedule that address problems before they result in failure, but without losing valuable working life. Or as ABB says of its predictive maintenance solution:10 “By pulling together previously disparate condition data from various assets, such as valves, switches, sensors, motors, switchgear, gearboxes, crushers and conveyors to collectively analyze and compare all data, [predictive maintenance] is able to provide forewarning of a potential fault (i.e., lubrication failure) with a proposed solution – all with enough time to address it before production is affected.”
Implementing Predictive Maintenance
It is only in recent years the technologies that underpin predictive maintenance have become both available and inexpensive enough be widely accessible. As Deloitte pointed out,11 “The decreasing cost of sensors, computing power, and bandwidth, coupled with increasing technological advancements, has made predictive maintenance a much more viable option, and one that could be feasible to scale at an enterprise-wide level.”
As a result, maintenance managers can now “gather data rapidly from connected machines via diverse sources […] enabling them to identify root causes that may have gone unnoticed before,” said Deloitte. It is this interconnectivity of data that gives predictive maintenance its power to address that central challenge of maintenance: getting the right part to the right place at the right time. “By driving those processes from real-world data, not guesses, about individual parts, predictive maintenance can help determine the optimal efficiency for most maintenance use cases.”
“The industry is developing capability in using these data to analyse patterns, identify value drivers, and predict failure to greater degrees of certainty than before,” agreed independent consulting firm Baringa.12 Meanwhile, “mobile technology and devices mean we can now access information outside the control room. Handheld devices, mobile cameras and visual aide tools, can take asset information to where operators need it most.”
It is, however, not enough to collect data: it also has to be accurate, as Baringa continued. “We find many organisations have not undertaken an asset audit for many years, and without true custodianship, existing data varies in degree of quality. Companies can start small by focusing on data quality of business-critical assets first.”
On top of data quality, other data-related challenges include determining the right health value threshold from the range of signal tags, ensuring data-collection equipment can survive the harsh mining environment and managing data transmission.
“Typically, it is not feasible to transmit all the raw data back to a central remote monitoring center,” explained David Siegel of Predictronics, a provider of AI-based predictive analytics solutions.13 “Local data storage and onboard edge analytics are needed to initially capture and process the data. This processed data is then typically sent back to a remote monitoring system [where] more advanced machine learning and pattern recognition analytics are conducted.”
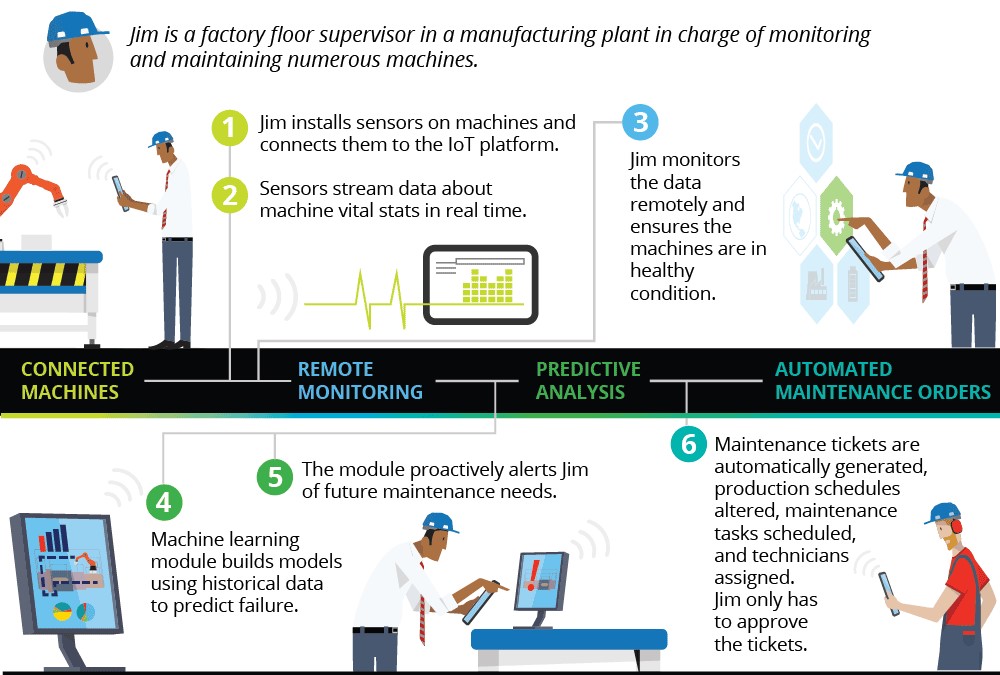
Nothing Without Context
While data provides the basis for predictive maintenance, it is not all that useful without context. Or, as Baringa put it: “data is not information”; turning data into information is therefore the next key step in implementing predictive maintenance.
One way to do this is to provide context, as OSIsoft explained:14 “Sensors may collect data that indicates a machine has stopped running. But that data does not automatically include the context of events – did the equipment stop running due to failure or due to the activation of an emergency stop button? This kind of context makes data meaningful. Context can help an analyst decide whether to treat that data point as part of a pattern predicting machine failure or as an extraneous event.”
The outset of establishing a predictive maintenance strategy may involve “a significant effort in data cleansing and mapping to events to enable effective analysis,” agreed Deloitte.15 “On an ongoing basis, it may require workers to adopt new practices […] Once gathered, these data can be aggregated across multiple assets and locations, stored, and analyzed by algorithms to predict failures and outcomes.
“It is therefore also considered important to choose the most appropriate algorithms and leverage machine learning to produce a predictive result. With this in mind, choosing and maintaining the software tools capable of this type of analysis is likely to be crucial to predictive maintenance success.”
Other issues that should be considered when assessing the shift to predictive maintenance include:14
- Security: interconnectivity brings risks as well as rewards. A proactive approach to cybersecurity and safeguarding critical assets should go alongside any strategy that involves digital and connected solutions.
- The need for new skills and organizational approaches. Data scientists may need to work alongside reliability and maintenance engineers to develop the algorithms and models that lie behind predictive maintenance systems.
- Equipment upgrades: mining equipment often runs for decades. Upgrading or replacing equipment with smart assets may require significant investment; retrofitting unconnected assets can also lead to increased cyber risks.
The Benefits
The set-up may be laborious, but results are powerful. According to Deloitte, adopting a predictive maintenance strategy can reduce the time required to plan maintenance by 20%-50%, increase equipment uptime and availability by 10%-20%, and reduce overall maintenance costs by 5%-10%.16
In a useful 2018 blog, WESCO put some specific examples to these numbers, outlining where mines may be able to benefit most from predictive maintenance:17
- Dragline excavators and electric rope shovels: monitoring vibration, electrical data and temperature profiles of major components can help to identify faults before they happen, allowing necessary maintenance to be planned and performed with minimum disruption.
- Conveyors: monitoring the load of each conveyor motor and controlling speed with variable speed drives not only helps to avoid unplanned downtime, but can extend the mechanical lifespan of the system.
- Ventilation systems: sensors on the ventilation system motors monitor for high vibration conditions and allow the system to react appropriately. In addition to keeping operators and equipment safe, monitoring ventilation systems can also help cut costs by reducing energy consumption and prolonging lifespans of other systems.
- Longwall mining equipment: hydraulic jacks, shearers, armored face conveyors, plow systems and other equipment used in longwall mining applications are all critical. If even one piece of equipment fails, the entire operation could grind to a halt, resulting in millions of lost potential. Monitoring the status of each piece of equipment can help avoid failures and disastrous shutdowns.
- Trucks: collecting information on a variety of factors like the load weight, fuel consumption, tire temperature and pressure, and total hours of operation allows for wear analysis and predictive maintenance. This visibility allows leaders to schedule maintenance during periods of lower production, to understand how the vehicles are being used and to make adjustments to best utilize the equipment.
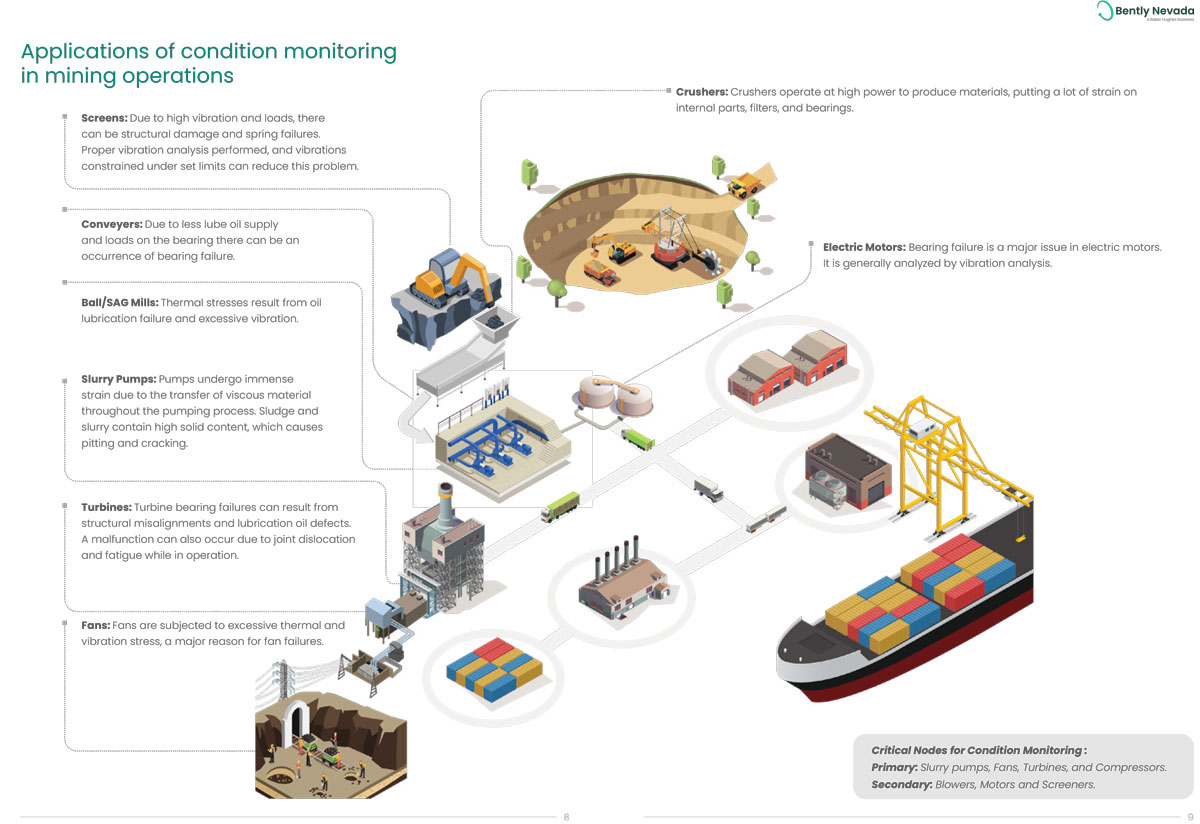
A New Standard for Maintenance
Predictive maintenance is a powerful opportunity for the mining industry to cut costs and improve productivity at a critical point in the industry’s development. It also fits into a pattern of asset management best practice that is emerging under international standard ISO 55000. First published in 2014, an update to this standard is now in the preparatory stages of development.
One of the benefits of ISO 55000 is that it provides a potentially helpful tool when assessing the relative merits of the various technologies and solutions now on the market. “The industry is getting flooded with new kinds of asset management tools that can exploit this wealth of information by, for example, enhancing predictive maintenance activities,” wrote GE’s David Appleyard of the power sector – but it is no less true of mining.18
“The key is to determine how these tools fit into an individual plant’s strategy and the overall corporate asset management policy. These two elements are complimentary, and it makes sense to have a guide like ISO 55000 to formalize how to use these tools to manage risk, cost, and regulatory considerations,” he said.
It also supports asset managers when it comes to rolling out new asset management programmes, such as predictive maintenance strategies. “As an asset manager, the first thing to consider during implementation is which assets should be included under the certification regime,” concluded Appleyard. “After making that decision, the asset manager can use ISO 55000 to establish a framework that formally maps elements such as mandated requirements, overall policy goals, and key objectives to the management strategy. In addition, with the advent of so many new digital asset management solutions, implementing an ISO 55000 program helps ensure that these solutions are consistent with the company’s overall objectives and ROI concerns.”
Prescriptive Maintenance: The Future
Predictive maintenance may be as far as we can go with the technologies available today. But it is not the end. To return to the five approaches of maintenance outlined earlier, the future – according to OSIsoft at least – is prescriptive maintenance.19
“The ultimate level of maintenance, prescriptive maintenance, involves the integration of big data, analytics, machine learning and artificial intelligence. [It] takes predictive maintenance a step further by automatically implementing an action to solve an impending issue, rather than recommending an action that is then carried out by operators […] A prescriptive maintenance system will be a cognitive system; it will have the ability to ‘think’ and can only perform at this level when there is interoperability between analytic systems and assets. This is the maintenance system of the future: the heady end goal of Industry 4.0.”
References
1. 2023: The Near Future of Mining, BDO (2019).
2. Leugner, L.T., ‘Same same but different – Mining equipment reliability and maintenance’, Machinery and Equipment MRO (7 May 2017): https://www.mromagazine.com/features/same-same-but-different-mining-equipment-reliability-and-maintenance-in-the-mining-sector/
3. Provencher, M., ‘Moving toward mining asset predictive maintenance’, OSIsoft (2020): https://www.osisoft.com/blog/moving-toward-mining-asset-predictive-maintenance
4. How leading mining companies are getting smarter with condition monitoring: Using proactive asset reliability to improve operations and profitability, Bently Nevada (2021), p. 7.
5. Much of the following discussion draws on The Digital Plant: A Four-Step Approach to Predictive Maintenance 4.0, OSIsoft (2020): https://explore.osisoft.com/metals-mining-materials-general-info/wp-thedigitalplant-a
6. Coleman, C., Damodaran, S., Chandramouli, M., and Deuel, E., ‘Making maintenance smarter: Predictive maintenance and the digital supply network’, Deloitte (09 May 2017): https://www2.deloitte.com/us/en/insights/focus/industry-4-0/using-predictive-technologies-for-asset-maintenance.html
7. OSIsoft, 2020.
8. What is Condition-based Maintenance? How CbM Works and When to Use It, FTMaintenance (22 January 2020): https://ftmaintenance.com/maintenance-management/what-is-condition-based-maintenance
9. Provencher, 2020.
10. ABB Ability™ Predictive Maintenance for mining: https://new.abb.com/mining/services/advanced-digital-services/predictive-maintenance-mining
11. Coleman, et al., 2017.
12. Data analytics in asset management for the mining industry, Baringa (2018): https://www.baringa.com/BaringaWebsite/media/BaringaMedia/PDF/Data-Analytics-in-Asset-Management-for-the-Mining-Industry.pdf
13. Siegel, D., ‘Predictive maintenance for mining vehicle applications’, Pumps & Systems (24 September 2021): https://www.pumpsandsystems.com/predictive-maintenance-mining-vehicle-applications
14. OSIsoft, 2020.
15. Coleman, et al., 2017.
16. Coleman, et al., 2017.
17. Predictive maintenance and analytics in mining, WESCO (2018): https://blog.wesco.com/predictive-maintenance-and-analytics-in-mining
18. Appleyard, D., ‘How to implement ISO 55000: Developing a strategic asset management plant’, GE (09 May 2017): https://www.ge.com/power/transform/article.transform.articles.2017.may.how-to-implement-iso-55000-dev
19. OSIsoft, 2020.